What Is Multi-Agent AI System & Why It Matters in Retail?
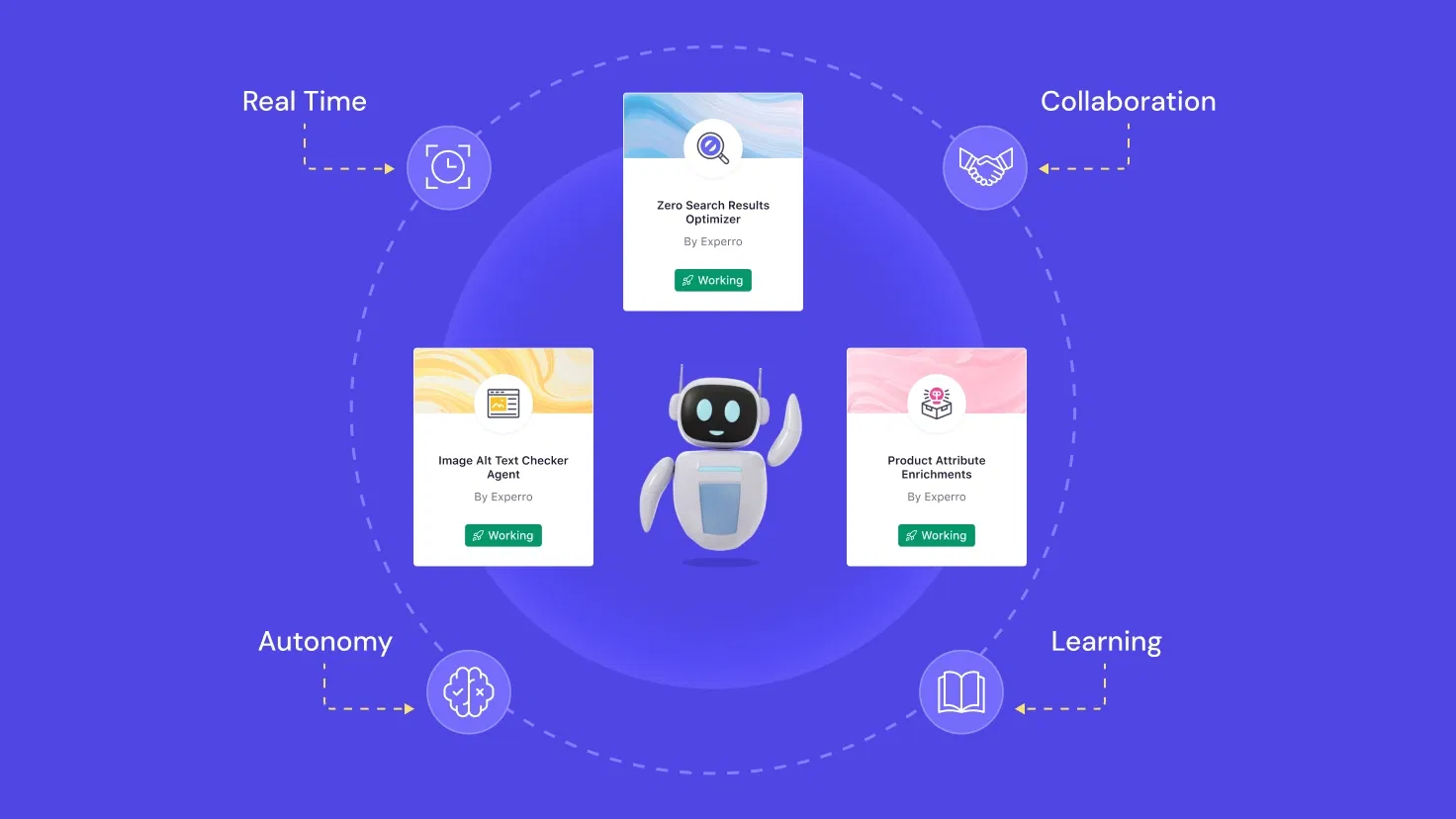
What’s Inside
- What is a Multi-Agent AI?
- How Does a Multi-Agent System Work?
- When Do Single AI Agents Reach Their Limits?
- Difference Between Multi-Agent Systems vs Single AI Agents
- What Are the Benefits of Multi-Agent Systems?
- What Are the Behaviors of Multi-Agent Systems?
- What Are Real-time Multi-Agent AI Use Cases?
- How Experro Helps You Build Smarter Workflows with Agentic Capabilities?
- Conclusion
Key Takeaways
- Multi-agent AI breaks down eCommerce workflows into specialized tasks like personalized search, pricing optimization, and inventory updates—all handled simultaneously for faster operations.
- They enable autonomous, context-aware decision-making, reducing reliance on human input while adapting to real-time user behavior.
- Multi-agent systems support scalability, agility, and continuous optimization, making them more effective than single-agent setups.
- Experro enables agentic workflows through modular architecture and intelligent orchestration of specialized AI agents.
Hundreds of personalized experiences, live pricing updates, real-time inventory sync, and campaign testing — all happening at once.
Not because one AI agent is doing it all, but because dozens of specialized multi-agent AI are working in parallel.
That’s the power of multi-agent artificial intelligence in eCommerce.
Unlike single-agent systems that handle one task at a time, multi-agent architectures assign specialized roles to each AI — search, content, pricing, analytics — allowing them to act simultaneously, communicate, and adapt.
The result? A faster, more intelligent eCommerce experience that scales effortlessly.
What is a Multi-Agent AI?
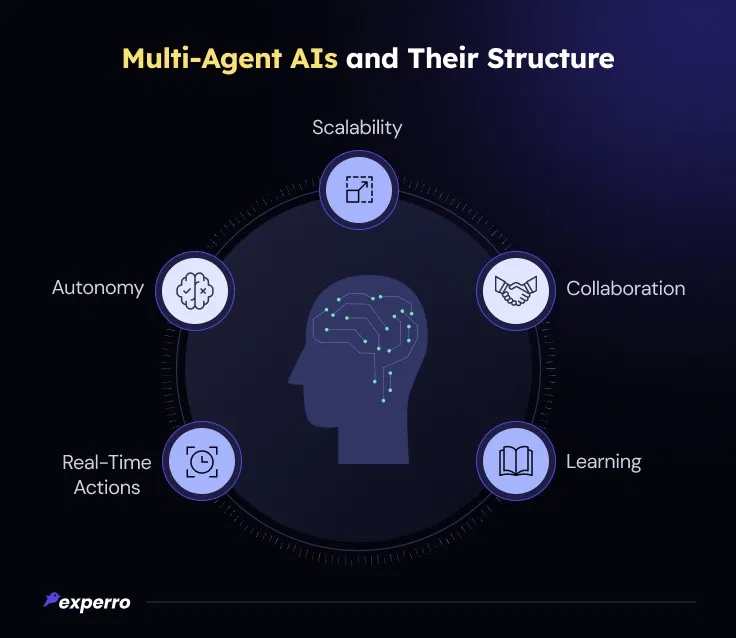
Multi-agent AI is a system composed of multiple intelligent agents that interact with each other and their dynamic environment to achieve individual or collective goals.
In simpler terms, it's like working with several AIs—each specialized—working together to solve complex tasks. These multi-agent AI systems divide the work and operate in sync to boost efficiency and speed.
The agents can be either collaborative or competitive depending on the task. This setup is different from traditional AI systems that rely on a single model to perform all tasks.
Multi AI agent architectures are being explored to boost performance, scalability, and adaptability across industries.
How Does a Multi-Agent System Work?
A multi-agent system in AI functions through distributed control. Each AI agent is autonomous and handles specific tasks.
Distinct agents operate in parallel, interact with one another, and make decisions based on shared data. Communication protocols are key—they enable the exchange of insights between agents.
The system can be decentralized, allowing for real-time reactions and faster outputs. In a multi-agent AI framework, tasks are split intelligently and executed with minimal dependencies.
Multi-agent LLMs (large language models) help businesses optimize for hyper-personalization and even scale eCommerce operations.
When Do Single AI Agents Reach Their Limits?
A single AI agent can be powerful—but only up to a point. As tasks grow more complex, a single agent-based system struggles with overload and limited contextual understanding.
It may fail to deliver when required to handle multi-step operations or real-time personalization across different touchpoints.
Multi-agent AI systems fill this gap by dividing work across multiple specialized agents. Multi-agent AI systems handle tasks like real-time inventory updates and omnichannel personalization by enabling fast, personalized, and coordinated decision-making.
Multi-agent AI systems fill this gap by dividing tasks across specialized agents—ideal for scenarios like real-time inventory updates or omnichannel personalization, where simultaneous, personalized, and coordinated decision-making is essential.
In single vs multi-agent AI, the latter clearly excels in flexibility and responsiveness. When scalability, efficiency, and data diversity are in play, the single-agent model hits a ceiling.
Let's know the major differences between single agent vs multi-agent in AI from the next section.
Difference Between Multi-Agent Systems vs Single AI Agents
While multi-agent systems and single AI agent systems aim to solve coordination complexity problems, they approach them in different ways.
Here's how they compare:
Basis of Distinction | Single AI Agent | Multi-Agent AI System |
---|---|---|
Control | Centralized | Decentralized |
Scalability | Limited | High (via multiple agents) |
Task Management | Linear – one task at a time | Parallel – multiple tasks run simultaneously |
Adaptability | Slower in adapting to manual updates | Fast, responsive via collaboration |
Use Case Fit | Simple or isolated tasks | Complex, dynamic, and multi-step environments |
System Behavior | Predictable | Emergent, evolving |
Common Examples of Use | Chatbots, simple recommendations | Smart search, supply chain management, etc. |
What Are the Benefits of Multi-Agent Systems?
In a digital-first world, businesses are always looking for faster, smarter, and more scalable solutions. A multi-agent AI system offers exactly that.
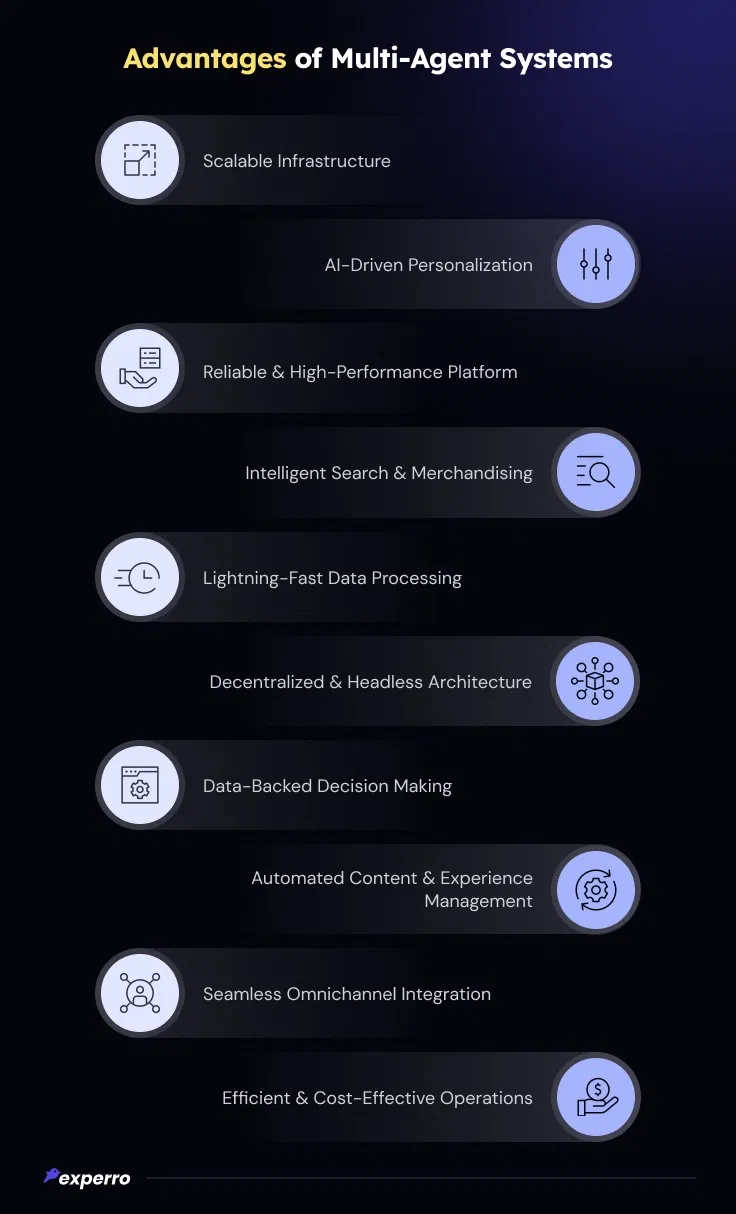
With its multi-agent orchestration, brands can streamline workflows and experience the benefits of improved efficiency and performance. Let’s explore how.
1. Scalability for Growing Businesses
A multi-agent system in AI enables you to scale without overloading a single model. Each multi-AI agent handles a dedicated task—like product attributes, dynamic pricing, or product tagging.
Whether you’re handling 10 users or 10,000, the overall system remains stable. It’s a future-ready architecture built to grow with your business.
2. AI-Driven Personalization for Better Customer Experiences
Multi-agent generative AI systems can power more personalized experiences. One agent analyzes customer behavior, another selects the right content, while another recommends the best-fit products.
This real-time coordination ensures users get content tailored to their exact needs. It’s like having a personalized concierge for every shopper. This setup enables multi-agent AI workflows that are fast, efficient, and accurate.
3. Increased Reliability and System Performance
With multiple AI agents working in sync, downtime is minimized. Parallel execution removes delays from waiting on separate agents’ outputs—making processes faster and more efficient.
If one agent fails, its issue doesn’t affect others—this means ensuring localized failures don’t bring down the entire platform. This isolation boosts reliability and helps maintain a seamless experience.
This multi-agent AI deployment ensures your customer experience is uninterrupted. It also allows for faster troubleshooting since issues are isolated from separate agents.
4. Intelligent Search & Better Merchandising Outcomes
In multi-agent AI in eCommerce, one agent may analyze user’s collective behavior, while another adjusts product visibility in real-time.
Together, they enhance content discoverability and relevance. This results in a smoother journey from search to checkout. Multi-agent collaboration in search also supports error correction, synonyms, and contextual relevance.
5. Rapid Insights Through Real-Time Data Processing
Multi-agent gen AI systems divide and conquer data tasks. One agent pulls data, another cleans it, and a third one processes it.
This division of labor speeds up operations and improves decision-making. In the world of real-time commerce, faster data means faster insights—and faster results.
6. Greater Flexibility with Headless Architecture
In a multi-agent AI framework, an independent agent can function separately. This decentralization supports headless architecture, where backend logic and frontend experiences stay loosely coupled.
It improves performance and allows businesses to integrate across platforms easily. It’s flexible, modular, and future-focused.
7. Faster, Smarter Decisions with Data at the Core
A multi-agent environment in AI enables better analytics. Each agent can track specific metrics—product views, shopping cart drops, bounce rates, cart abandonment recovery etc.
These insights are then combined to form a complete customer journey map. The result? More agents with informed strategies and measurable ROI. It turns data into action.
8. Effortless Content and Experience Automation
Multi-agent conversational AI can manage everything from banners to blogs. One agent could generate content, another could A/B test it, and a third could analyze engagement.
This level of automation boosts marketing efficiency and speeds up content cycles and intelligent customer journey orchestration at scale.
9. Unified Experiences Across All Channels
With the help of multi-AI agents powered by agentic AI, businesses can work across web, mobile, in-store, and email. Each channel is powered by a dedicated AI agent that understands user behavior specific to that platform.
Omnichannel eCommerce ensures to create consistent experiences everywhere. This is key to delivering a unified brand presence.
10. Reduced Costs and Streamlined Operations
Businesses reduce waste and cut costs by optimizing each task with the right AI agent. No need to over-rely on a large single AI model.
Multi-agent generative AI solutions can be deployed modularly, allowing for better budget control. You get more done, for less.
Now that we have learned about the benefits of multi-agent systems, let’s learn about different behaviors of these.
What Are the Behaviors of Multi-Agent Systems?
Understanding how multi-agent AI systems behave is key to unlocking their full potential. These systems don’t just perform isolated tasks—they learn, collaborate, and evolve over time.
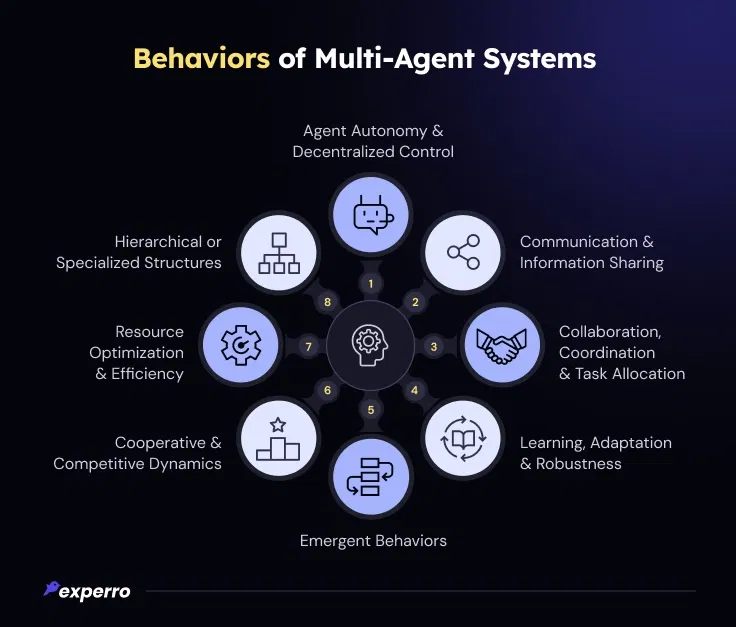
In a multi-agent AI framework, behaviors emerge from constant interaction among agents. From autonomy to coordination, every agent plays a unique role in the system.
Let’s explore some of the defining behaviors of multi-agent systems in AI.
1. Agent Autonomy & Decentralized Control
Each agent in a multi-agent system operates independently. It makes decisions without needing approval from a central unit. This autonomous multi-agent system behavior allows the platform to be resilient and agile.
Decentralized control ensures that even if one agent fails, others continue operating. This structure also makes multi-agent AI deployment more scalable and fault-tolerant.
For example, in an online store, if the pricing agent crashes during a flash sale, the recommendation and inventory agents still keep running, ensuring the customer journey isn’t disrupted.
2. Communication & Information Sharing
Multiple interacting intelligent agents constantly share data. They use structured communication protocols to stay aligned.
This seamless multi-agent collaboration improves accuracy and coherence across all tasks. Conversational commerce is the core of a successful multi-agent AI system.
For example, one agent might share inventory updates, while another responds by adjusting promotions on the leftover inventory.
3. Collaboration, Coordination & Task Allocation
In multi-agent orchestration, each agent is assigned a specific role based on its strengths. They work together to complete complex workflows—like onboarding, personalized journeys, or A/B testing.
Tasks are distributed intelligently, making operations faster and more efficient. This dynamic coordination is a key reason why multi-agent generative AI in eCommerce outperforms traditional systems.
For example, a customer opens the app—one agent personalizes product suggestions, another triggers a welcome email flow, while a third ensures inventory availability for recommended items.
4. Learning, Adaptation & Robustness
Agents don’t just follow instructions—they learn. A multi-AI agent system setup allows agents to observe outcomes and adjust their behavior as per the eCommerce analytics.
Over time, they become more accurate and efficient. This learning and adaptation make the system more robust and intelligent. It also helps in building long-term strategies based on real-time insights.
For example, if customers frequently ignore a certain discount, the pricing agent learns to test alternative offers based on past engagement patterns.
5. Emergent Behaviors
Sometimes, unexpected but valuable behaviors emerge from agent interactions.
Through coordinated decision-making, LLM in eCommerce can exhibit emergent behaviors that push innovation forward, even beyond what businesses initially plan.
For example, agents trained for personalization may unintentionally optimize for upselling too.
6. Cooperative & Competitive Dynamics
In some cases, agents cooperate to achieve a common goal. In others, they compete to deliver the best outcomes—like content variations in multi-agent A/B testing.
This mix of cooperation and competition boosts innovation. It also helps optimize strategies across different departments or customer experience KPIs.
For example, a content generation agent creates two separate product page versions. The system runs A/B tests and retains the version with better engagement.
7. Resource Optimization & Efficiency
In a multi-agent AI architecture, tasks are not duplicated. Agents use shared resources smartly.
This minimizes waste and maximizes processing speed, making it ideal for eCommerce operations.
For instance, instead of processing the same dataset multiple times, one agent can prep the data and others can reuse the same set of data.
8. Hierarchical or Specialized Structures
Some multi-agent gen AI systems use a hierarchy. A master agent may oversee decision-making, while others perform specialized tasks.
This setup creates structured workflows and reduces chaos. Hierarchical models also make multi-agent AI workflows easier to manage and debug when scaling up.
For example, a lead agent monitors checkout performance. If abandonment spikes, it triggers other agents to tweak recommendations, delivery options, or support prompts in real time.
Now that we have learned about multiple behaviors of AI agents, let’s dive deeper into their real-time use cases.
What Are Real-time Multi-Agent AI Use Cases?
The real magic of multi-agent AI systems lies in their applications. From eCommerce to security, these systems are solving problems that single-agent AI just can’t handle.
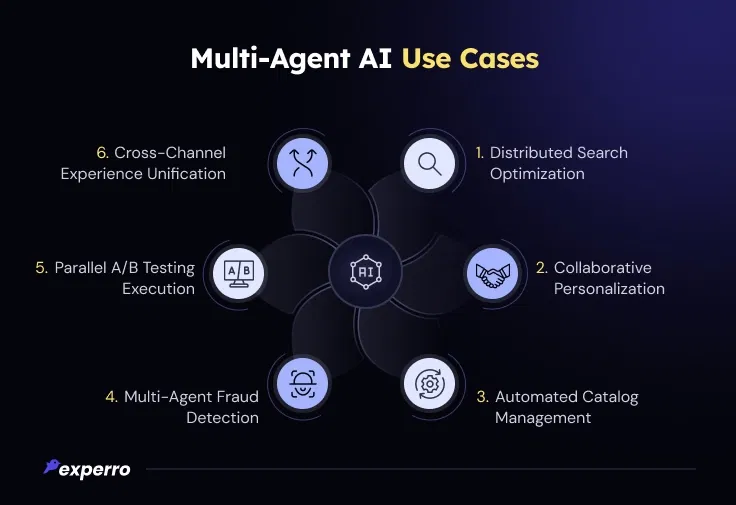
Businesses exploring the difference between single agent and multi-agent in AI will find major value in these advanced reasoning capabilities.
Let’s dive into some practical, high-impact multi-agent AI use cases.
1. Distributed Search Optimization
In an AI multi-agent shopping system, one agent may focus on query correction, others on filtering, and ranking.
Together, they refine results instantly. This leads to better product visibility and faster response times.
Distributed search is a core capability of multi-agent systems AI platforms, especially in high-traffic eCommerce environments.
2. Collaborative Personalization
Multi-agent conversational AI can be used to deliver real-time, hyper-personalized experiences. One agent tracks user behavior, another recommends products, and the third adjusts layouts dynamically.
The result is a unified, tailored journey that feels personal and relevant. Multi-modal AI agents also contribute by adapting to multimodal search formats—text, image, or voice.
3. Automated Catalog Management
A multi-agent AI chatbot can help your e-store with catalog management, while another handles SEO metadata and another monitors out-of-stock alerts.
These tasks are typically siloed in traditional systems. But with multi-agent AI orchestration, everything is automated and updated in real-time. This reduces manual effort and improves data consistency.
4. Multi-Agent Fraud Detection
Fraud detection is no longer a one-size-fits-all task. One agent monitors payment behavior, another tracks IP and location data, and a third evaluates transaction speed.
Together, these agents detect anomalies faster. A multi-agent environment in AI makes fraud detection more proactive and precise.
5. Parallel A/B Testing Execution
Why run one test at a time when you can run too many tools in parallel?
A multi-agent AI system allows different agents to test product images, CTAs, layouts, and pricing—all simultaneously.
The results are collected faster, and decision-making becomes easier. Parallel testing is a perfect example of how multi-step AI agents can speed up growth.
6. Cross-Channel Experience Unification
In omnichannel retail, one agent can manage email campaigns, another in-app messages, and various types of website personalization.
These agents coordinate to ensure consistency. This multi-agent AI coordination helps brands create unified customer experiences across all digital touchpoints.
Nearly 48.9% of U.S. retail companies leverage AI for marketing automation, enhancing their ability to engage customers effectively.
How Experro Helps You Build Smarter Workflows with Agentic Capabilities?
Experro helps you build smarter, more adaptive workflows through powerful agentic experience capabilities.
The platform enables autonomous decision-making, intelligent content delivery, and dynamic experience orchestration—all without manual intervention.
From real-time search personalization to automated eCommerce merchandising and seamless omnichannel coordination, every component works in sync to deliver precision at scale.
Experro's modular architecture supports decentralized execution, making your systems faster, more responsive, and easier to scale.
With built-in DXP analytics and automation, your workflows become self-optimizing over time. Experro doesn’t just support agentic experiences—it elevates them into a strategic advantage for your business.
Conclusion
The evolution from single-agent AI to multi-agent AI systems is more than a technical upgrade—it's a strategic leap.
With scalable, decentralized, and intelligent agent networks, businesses can streamline workflows, personalize experiences, and optimize performance across channels.
Whether you're managing large-scale operations or delivering dynamic digital experiences, the multi-agent AI framework unlocks new levels of efficiency and intelligence.
The future of AI isn't about one agent doing everything—it's about many agents working smarter, together.
Ready to build an experience that evolves with AI? Let’s talk on a call.
FAQs
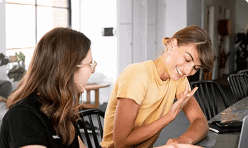
Pallavi Dadhich
09 April 2025Pallavi is an ambitious author known for her expertise in crafting compelling content across various domains. Beyond her professional pursuits, Pallavi is deeply passionate about continuous learning, often immersing herself in the latest industry trends. When not weaving words, she dedicates her time to mastering graphic design.