Profile-based Recommendations to Enhance Personalization
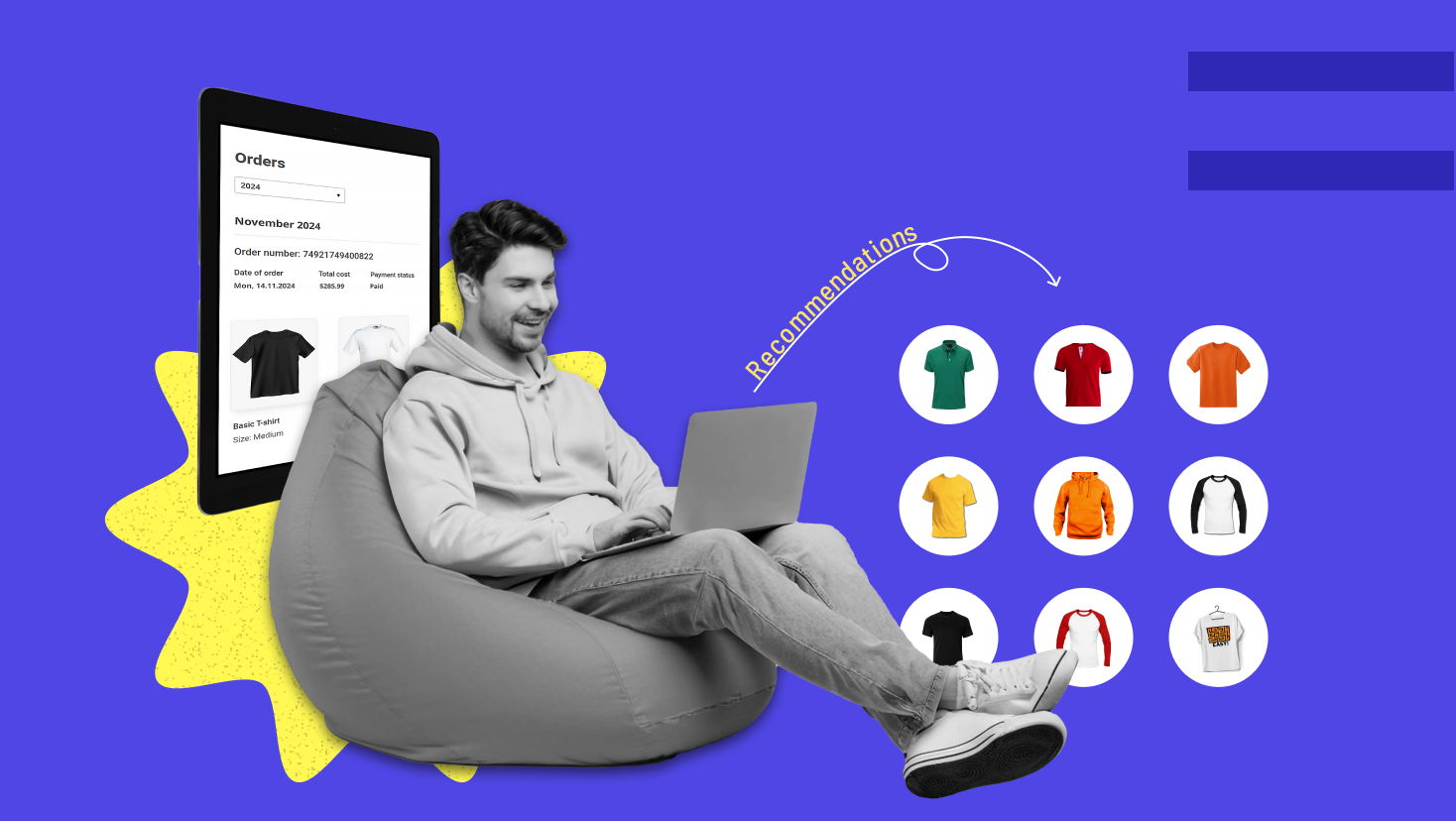
What’s Inside
- What Are Profile-Based Recommendations?
- How Do Profile-Based Recommendations Work?
- What Are the Types of Profile-Based Recommendations?
- What Are the Benefits of Profile-Based Recommendations?
- Challenges in Implementing Profile-Based Recommendations
- Achieve Precision in Profile-based Recommendations With Experro
- Conclusion
Key Takeaways
- Profile-based recommendations utilize user data to align content and products with individual preferences.
- Advanced AI and ML drive the effectiveness of recommendation systems in modern eCommerce.
- Combining multiple recommendation types (behavioral, demographic, preference-based & contextual) enhances personalization and optimizes customer journeys.
- Experro delivers game-changing personalization with real-time, scalable profile-based recommendations, setting a new benchmark in customer engagement.
Every customer journey is unique, yet businesses today are expected to deliver perfectly tailored experiences at every touchpoint.
This is where profile-based recommendation in eCommerce come into play.
Transforming raw data like browsing behavior, purchase patterns, and context into meaningful, personalized suggestions.
By leveraging advanced profile-based algorithms, businesses can not only meet but exceed customer expectations, driving satisfaction, engagement, and loyalty.
In this guide, we’ll delve into how these systems work and explore the various types of recommendations they offer.
We’ll also highlight their benefits and explain how companies can leverage them effectively to create lasting customer connections.
What Are Profile-Based Recommendations?
Profile-based recommendations are personalized suggestions generated by analyzing a shopper’s profile and preferences.
These recommendations are based on explicit data such as:
- browsing history
- purchase patterns
- demographics
- contextual information.
By understanding these patterns, businesses can deliver highly targeted suggestions tailored to individual customers or specific customer segments.
Profile-based recommendations enhance personalization and optimize digital merchandising by aligning product placement with user preferences.
With AI profile-based recommendation strategies, businesses can elevate their customer engagement and create memorable shopping experiences.
Now, let’s know more about the working of these recommendations.
How Do Profile-Based Recommendations Work?
A profile-based recommendation relies on a user profile recommendation engine to analyze and process user data.
Here's how it typically works:
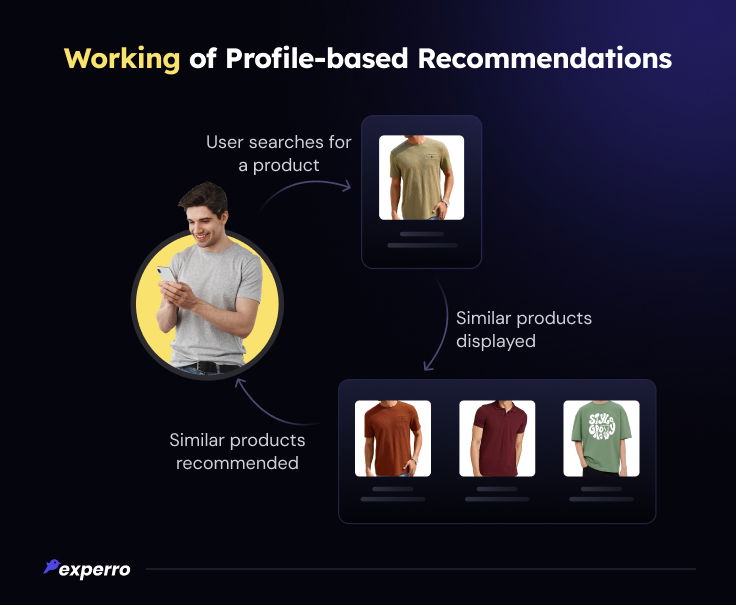
- Data Collection: The system gathers information from various sources, such as browsing behavior, purchase history, and contextual signals.
- Profile Building: A detailed profile is created for each user or product using advanced profile-based algorithms.
- Pattern Analysis: The system identifies trends and relationships within the relevant data using AI in profile-based recommendations.
- Recommendation Generation: Based on the profile, the system delivers personalized profile recommendations, or buy-it-again suggestions.
- Real-time Updates: As new data comes in, the system updates profiles dynamically to ensure real-time profile recommendations while continuously optimizing results through eCommerce analytics.
With Experro, your businesses can simplify these processes with an intuitive platform that leverages AI and machine learning for profile-based recommendation capabilities for scalable, real-time personalization.
What Are the Types of Profile-Based Recommendations?
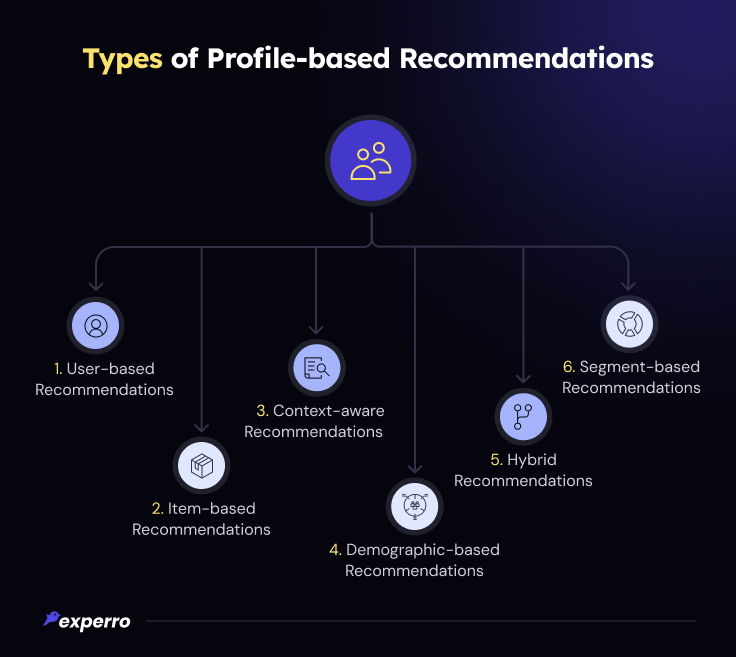
Here are the key types of profile-based recommendations:
1. User-based Recommendations
A user based recommendation system identifies users with similar preferences and suggests items based on what others in the group have liked or purchased.
This approach works well in communities with overlapping interests, such as streaming platforms or eCommerce websites.
It excels in delivering personalized profile recommendations by focusing on behavioral similarities.
For instance, if two users share similar browsing habits, one may receive recommendations influenced by the other’s activity.
2. Item-based Recommendations
This approach focuses on the relationships between items.
It suggests similar products that a user has interacted with, such as recommending accessories based on a purchased smartphone or offering buy-it-again recommendations for frequently used products.
Unlike user-based methods, it doesn’t rely on user-to-user similarities but instead emphasizes the attributes and associations of items.
This is especially useful for creating affinity-based recommendations where certain items are commonly purchased together.
For example, recommending a phone case when a customer buys a smartphone.
3. Context-aware Recommendations
Context-aware recommendations consider factors like time, location, and device type.
These suggestions dynamically adapt to user behavior and are enhanced by eCommerce filters for precise product discovery.
Context-aware systems are particularly impactful in industries like travel and retail, where timing and location can significantly influence purchasing decisions.
For example, the system might recommend warm clothing to users browsing from colder regions during winter.
4. Demographic-based Recommendations
Demographic-based recommendations group users by shared characteristics such as age, gender, or location.
This type of recommendation complements eCommerce searchandising efforts by aligning product displays with audience segments.
By aligning with the shopper's profile and preferences, businesses can better target broader audience categories.
For instance, suggesting trendy backpacks to college students or region-specific items to users in a particular area.
5. Hybrid Recommendations
Hybrid recommendations combine multiple methods, such as user-based and item-based approaches, to enhance accuracy.
This approach leverages the strengths of each method, minimizing their weaknesses, such as cold-start issues.
Hybrid systems are widely used in industries like eCommerce and entertainment, where advanced profile-based algorithms can refine personalization.
For example, the system might use user similarities to filter item recommendations, creating a balanced, more refined suggestion.
6. Segment-based Recommendations
Segment-based recommendations group users into predefined segments (such as "frequent buyers" or "bargain hunters") and suggest items relevant to those groups.
Unlike user-based systems, these operate on broader patterns rather than particular user preferences.
This type of recommendation excels in delivering results for businesses that lack granular user data, as it draws on collective trends within segments.
Focusing on profile type recommendations helps create tailored marketing campaigns and promotions for specific audience categories.
For example, a "frequent buyer" segment might receive recommendations for loyalty rewards, while "bargain hunters" are shown exclusive discounts.
What Are the Benefits of Profile-Based Recommendations?
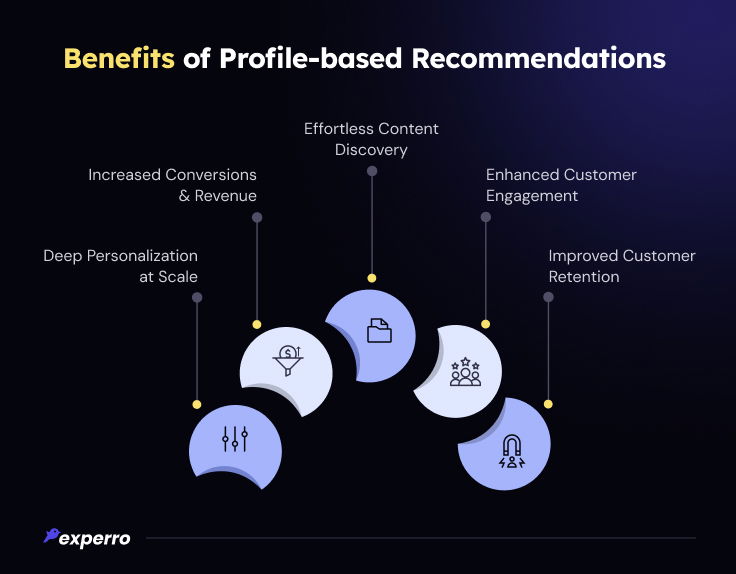
Profile-based recommendations leverage customer data to create tailored experiences that resonate with individual preferences, driving engagement and satisfaction.
Here are some key benefits of this approach:
1. Deep Personalization at Scale
AI profile-based recommendation strategies create unique customer experiences for thousands of users simultaneously.
By analyzing a user profile recommendation system, businesses can deliver personalized suggestions at a scale that resonate with individual needs.
This level of scalability ensures that every customer interaction feels tailored, whether it’s for a niche product or a trending item. The ability to personalize at this level boosts user satisfaction and brand loyalty.
2. Increased Conversions and Revenue
Relevant suggestions drive more purchases, turning casual browsers into loyal customers. Businesses using platforms like Magento can further optimize performance and improve product recommendations to drive conversions.
With a profile-based recommendation, businesses can showcase products that align closely with the shopper’s profile and preferences, creating a seamless shopping experience.
The buy-it-again recommendations not only increase the likelihood of a purchase but also encourage cross-selling and upselling, significantly impacting revenue growth.
3. Effortless Content Discovery
Simplifies navigation for users, helping them find products that meet their needs through improved content discovery.
A user profile recommendation engine organizes and presents options tailored to individual preferences, reducing the time users spend searching.
Features like view-it-again recommendations help customers rediscover items they showed interest in, making the shopping journey smoother. This enhanced discovery process improves overall user experience and satisfaction.
4. Enhances Customer Engagement
Tailored digital customer experience keeps customers engaged and reduce bounce rates.
With personalized profile recommendations, businesses can create meaningful interactions that resonate with users through email campaigns, in-app notifications, or website suggestions.
This keeps customers actively involved and increases their likelihood of exploring more products. Affinity-based recommendations also build a stronger connection with users by reflecting their unique preferences.
5. Improves Customer Retention
Satisfied customers are more likely to return, improving lifetime value. Profile-based recommendations in eCommerce ensure that users see relevant, timely suggestions that make them feel understood.
Retention is further supported by dynamic, real-time profile recommendations, which adapt to changes in user behavior or interests.
When customers consistently find value in their interactions, they are more likely to stay loyal to the brand.
Purchases involving a clicked recommendation see a 10% higher AOV and five times higher per-visit spend compared to those without recommendation interactions.
Challenges in Implementing Profile-Based Recommendations
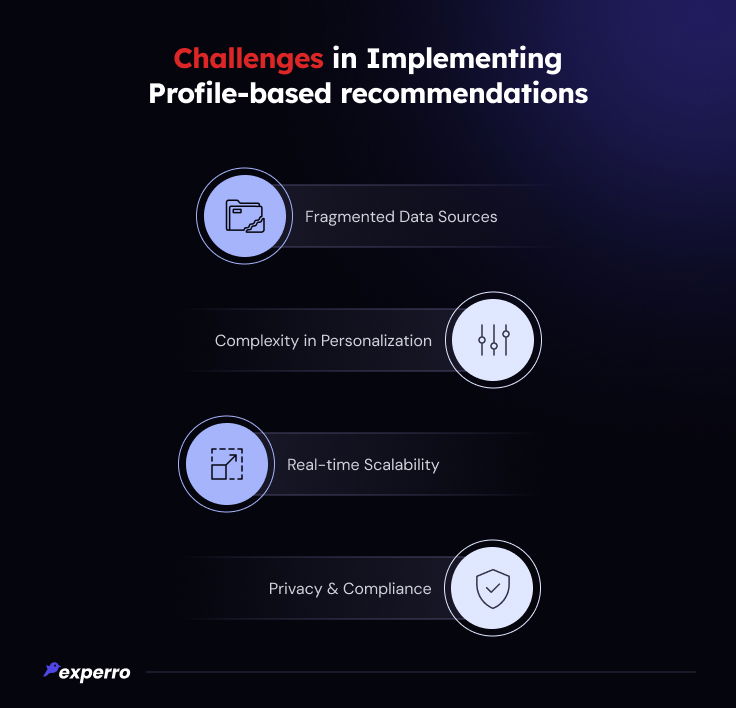
Implementing profile-based recommendations can transform customer experiences, but it comes with its own set of challenges that businesses need to address to ensure success:
1. Fragmented Data Sources
Data silos prevent the integration of information from multiple channels, making it difficult to create unified profiles.
This fragmentation limits the accuracy and relevance of AI profile-based recommendations. A centralized system is essential to bridge these data gaps.
Experro unifies data from all touchpoints into a seamless flow, enabling precise and actionable real-time profile recommendations.
2. Complexity in Personalization
Personalization requires advanced profile-based algorithms that can analyze diverse user behaviors and adapt to changing trends.
The lack of flexible tools often complicates the implementation of customer profile-based recommendation strategies.
Experro simplifies personalization with a no-code interface, making it easy to build and manage user profile recommendation systems.
3. Real-time Scalability
Handling millions of users and their interactions in real-time can strain systems, especially when managing features like predictive search for eCommerce.
System slowdowns or delays can impact customer experiences negatively.
Experro’s cloud-native architecture ensures robust, real-time profile recommendations without any compromise on speed or accuracy.
4. Privacy and Compliance
Protecting user data and ensuring compliance with privacy regulations is a significant challenge. Businesses must balance personalization with transparency and trust.
Experro adheres to global privacy standards, ensuring data security while delivering highly effective profile-based recommendations.
Achieve Precision in Profile-based Recommendations With Experro
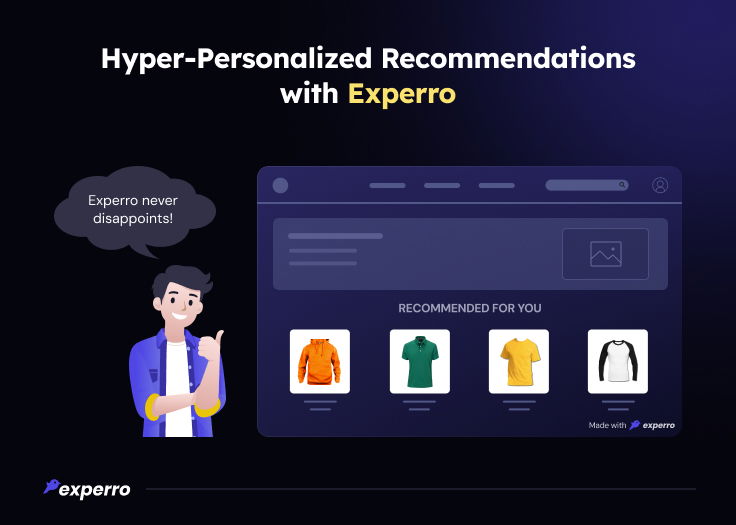
Want to put your customers in awe? Experro makes it effortless to deliver unforgettable, personalized experiences that keep them coming back.
The platform enables businesses to deliver all types of personalized eCommerce recommendations tailored to individual customer needs.
Unifying data from multiple sources provides a complete view of the customer, ensuring precision and scalability in personalization.
Experro combines advanced algorithms with hyper-personalization and seamless integration, making it easy to craft dynamic, engaging experiences at scale.
As a complete digital experience platform (DXP), Experro supports personalization across the entire customer journey, from discovery to conversion, while ensuring robust data security and compliance.
This makes Experro the ultimate solution for businesses looking to enhance engagement, increase conversions, and build lasting customer loyalty.
Conclusion
A profile-based recommendation is a game-changer for businesses aiming to deliver highly personalized experiences.
From enhancing user engagement to driving revenue growth, these recommender systems hold immense potential.
While challenges like data silos and scalability exist, solutions like Experro make implementing AI profile-based recommendation strategies seamless and efficient.
Ready to revolutionize your personalization strategies? Experro is here to help you unlock the full potential of profile-based recommendations in eCommerce.
For more information on profile-based recommendations, schedule a call with our experts.
FAQs
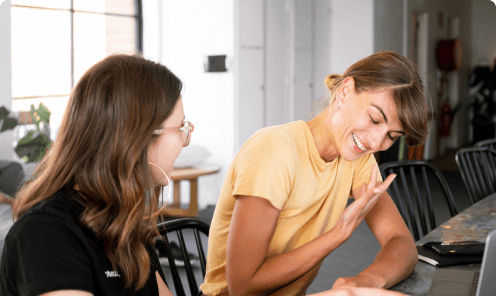
Pallavi Dadhich
13 December 2024Pallavi is an ambitious author known for her expertise in crafting compelling content across various domains. Beyond her professional pursuits, Pallavi is deeply passionate about continuous learning, often immersing herself in the latest industry trends. When not weaving words, she dedicates her time to mastering graphic design.