Multimodal Search: The eCommerce Game Changer of 2025!
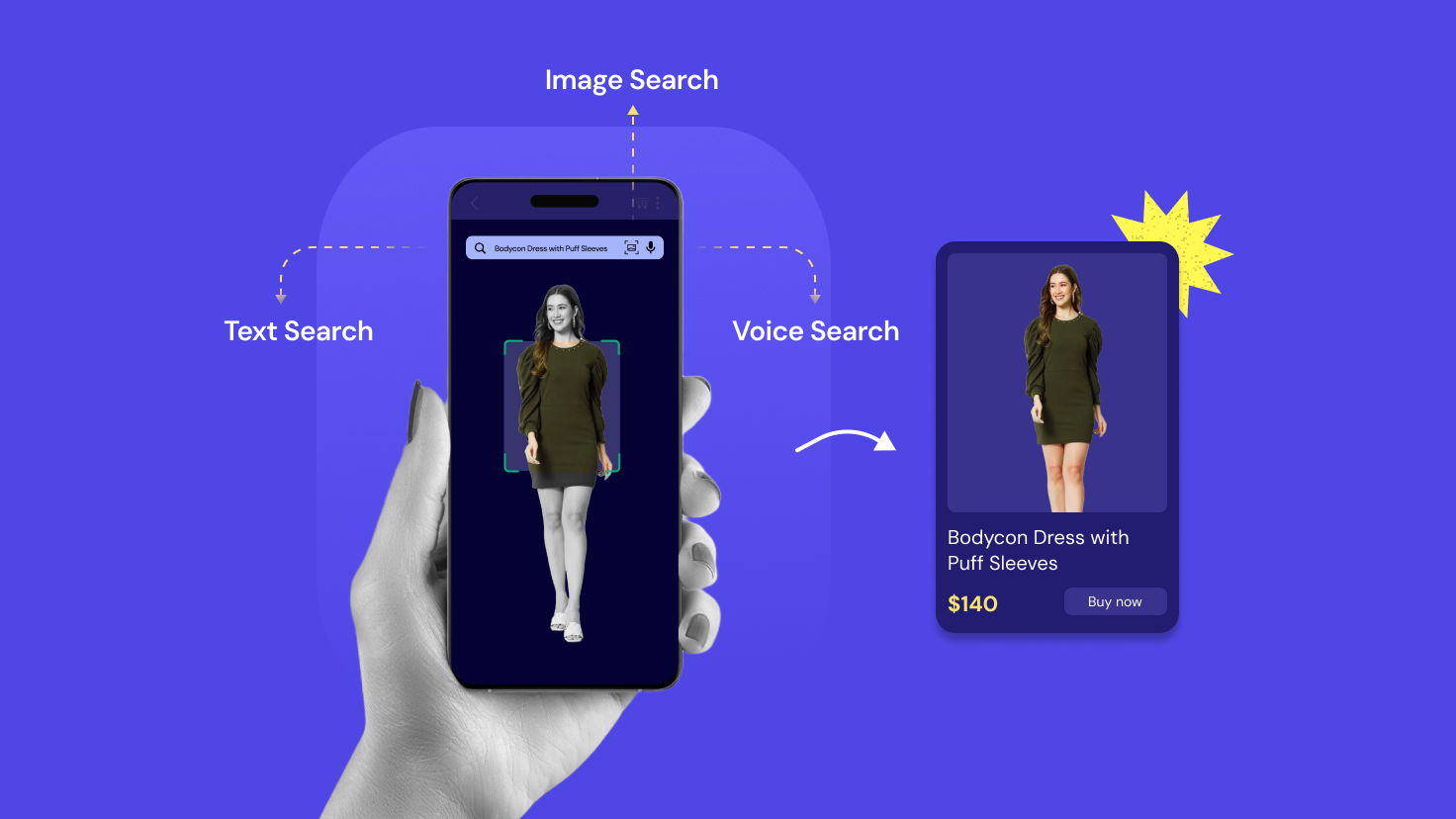
What’s Inside
- What Is Multimodal Search?
- What Are the Examples of a Multimodal Search?
- How Does Multimodal Search Work?
- How Does Multimodal Search Differ from Basic Search Functionality?
- What Are the Benefits of Multimodal Search in eCommerce?
- What Are the Use Cases of Multimodal Search in eCommerce?
- What Are the Key Challenges You Might Face in Adopting Multimodal Search?
- Transform Your eStore with Experro’s Next-Gen Multimodal Search Functionality!
- Conclusion
Key Takeaways
- Multimodal search in eCommerce allows customers to search using text, images, and voice, offering a more convenient shopping experience.
- Multimodal search technology is essential for eCommerce as it meets the growing demand for smarter, more intuitive product discovery.
- By analyzing context, multimodal search helps in delivering highly personalized, relevant results, driving customer satisfaction and loyalt
- Experro's AI-powered platform provides multimodal search integration capabilities for faster and more intuitive eCommerce experiences.
Imagine finding a product by simply describing it, uploading an image, or speaking—combining all these methods into one smooth, intuitive search experience.
This is the potential of a multimodal search bar. As eCommerce continues to evolve, customers demand smarter, more intuitive ways to find what they’re looking for.
Multimodal AI search optimization revolutionizes product discovery by blending various input methods for accurate and context-rich results.
Let’s explore the meaning of multimodal RAG and how it works.
What Is Multimodal Search?
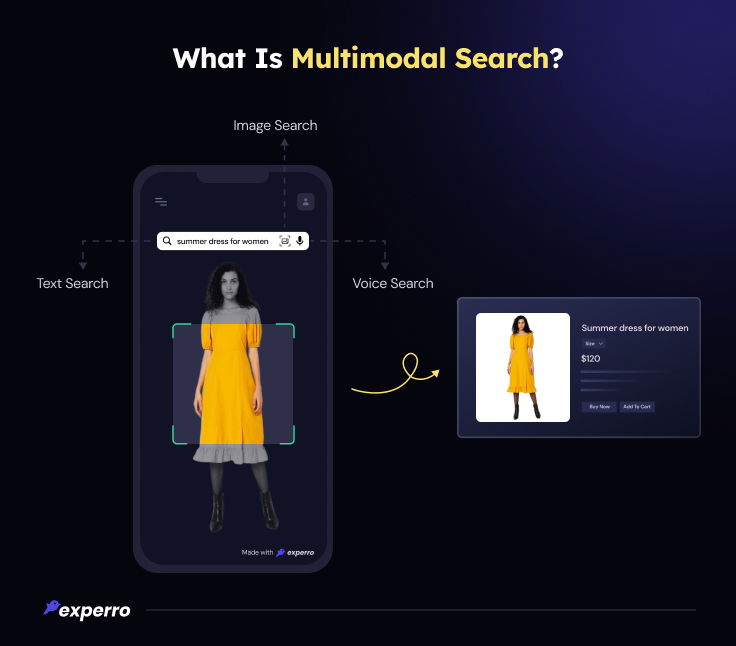
Multimodal search definition - It is a search technology that combines multiple input methods—such as text, voice, and images—to deliver precise search results.
Unlike traditional search, multimodal systems leverage advanced artificial intelligence and machine learning to process diverse inputs simultaneously.
For example, a user can upload a picture of a product and add text and image embeddings (attachments) for more details. This approach ensures better search relevance and accuracy.
It also bridges the gap between different customer preferences, creating a more inclusive shopping experience.
What Are the Examples of a Multimodal Search?
Multimodal AI search enhances product discovery by combining different input types for more accurate results.
The following multimodal search examples show how integrating text, image, and voice can streamline the shopping experience.
1. Searching for a Fashion Item Using Text and Image
A user looking for a specific dress can upload a reference image while describing details like "red floral maxi dress with puff sleeves".
The search engine processes both inputs, matching the visual style with the textual description to deliver highly relevant results.
This enhances accuracy, especially when product variations exist in different colors, patterns, or styles.
2. Finding a Jewelry Piece with Voice and Image
A customer searching for an engagement ring can upload a picture of a design they like while saying, "Show me similar rings with a rose gold band".
The system analyzes both inputs, considering design elements, metal type, and gemstone shape to refine the results.
This makes it easier for shoppers to find jewelry that matches their preferences without needing multiple searches.
How Does Multimodal Search Work?
eCommerce multimodal search integrates advanced technologies to process and interpret diverse inputs logically.
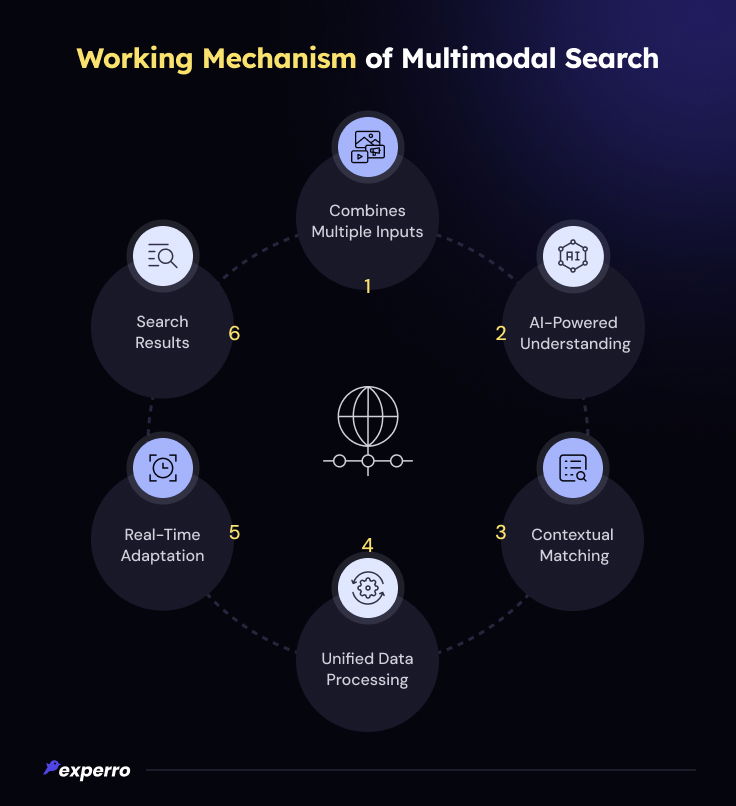
Here’s how it works:
1. Integrating Multiple Inputs
Multimodal vector search processes various inputs like text, images, and voice simultaneously. For instance, a customer can describe a product verbally while uploading a reference image to refine the search.
This synergy of inputs, with the help of fuzzy search, helps create a more accurate understanding of the query.
By leveraging multiple input methods like text, images, and voice, it adapts to different user preferences, ensuring both flexibility in search methods and precision in results.
2. Processing with Generative AI-Powered Understanding
Artificial Intelligence (AI) plays a pivotal role in interpreting inputs. It uses natural language search (NLS), image modalities, and voice recognition to understand the user’s intent.
Generative multimodal AI systems ensure that even vague or complex queries are handled efficiently. Furthermore, ML models continuously enhance the system’s ability to decode inputs over time.
This makes the search experience smarter and more intuitive, reducing the likelihood of irrelevant results.
3. Refining Results Through Contextual Matching
The system analyzes the context behind each input query to deliver neural search results. For example, it considers user preferences, past searches, and the relationships between the inputs to refine results further.
Multimodal semantic search ensures that the system doesn’t treat inputs in isolation, leading to more personalized results.
This capability is particularly valuable in eCommerce, where user context can significantly impact product recommendations and conversions.
4. Unified Data Processing
Multimodal product search engine operates on a unified platform that combines different data modalities. This eliminates silos and ensures that relevant information is processed holistically, enhancing accuracy and speed.
The unified data processing approach also simplifies backend operations, making it easier for businesses to manage and analyze large volumes of data.
This comprehensive processing ensures consistency in delivering results, no matter how complex or varied the inputs are.
5. Real-Time Adaptation
The system adapts to real-time inputs, dynamically updating search results as new information is added.
This ensures a fluid and interactive search experience for users. Real-time adaptation allows customers to refine their searches on the go, providing instant feedback and narrowing down options.
The agility in response makes the search journey more engaging and efficient, encouraging users to interact more with the platform.
6. Display Precise Search Results
Multimodal RAG delivers highly relevant results by combining AI-driven ranking, contextual analysis, and user intent recognition.
It intelligently filters and organizes results based on multiple input types, ensuring accuracy. Visual, textual, and voice-based queries are processed together to refine search outputs dynamically.
This precision helps users find the most relevant products faster, improving engagement and conversions.
How Does Multimodal Search Differ from Basic Search Functionality?
Unlike basic search, which typically relies on a single input type (only text), multimodal AI search processes multiple inputs simultaneously for precise results.
It is more intuitive and context-aware, delivering results that align closely with user intent.
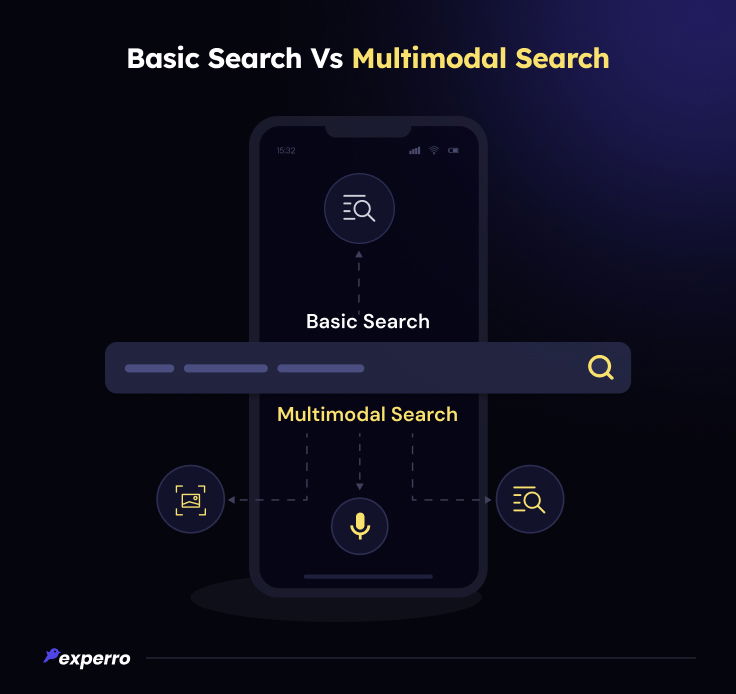
Below is a table highlighting the differences between basic text-based search functionality versus the multimodal search:
Feature | Basic Search | Multimodal Search |
---|---|---|
Input Type | Single input (usually text) | Multiple inputs (text, image, voice, etc.) |
Context Awareness | Limited context, based on keywords | Context-aware, processes multiple factors |
Result Accuracy | Matches based on keywords only | Matches based on richer data inputs |
User Experience | Static, one-dimensional | Dynamic, personalized & interactive |
Flexibility | Limited to text queries only | Supports diverse search methods (text, image, voice) |
What Are the Benefits of Multimodal Search in eCommerce?
Leveraging large language models for multimodal search brings transformative benefits to eCommerce platforms, creating value for both businesses and customers.
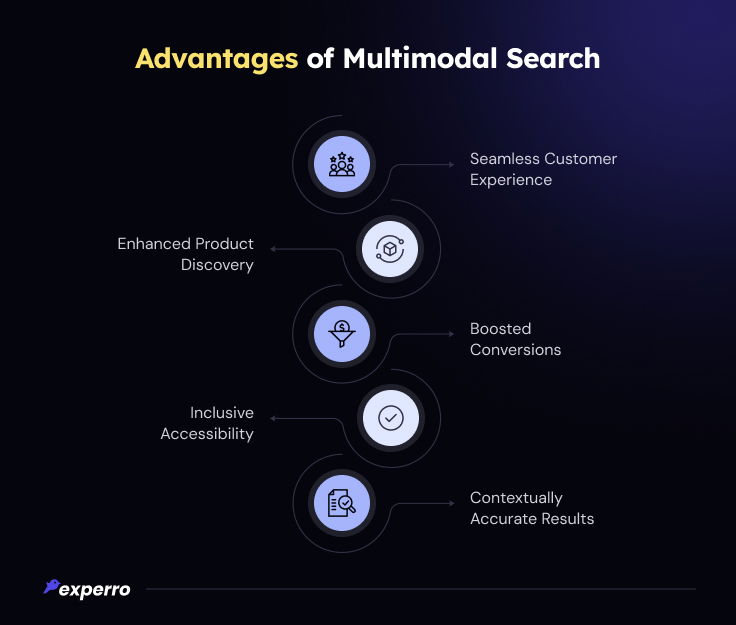
Let's explore the advantages in detail:
1. Seamless Customer Experience
By combining different modalities and input methods, eCommerce facilitates multimodal AI search that caters to varying customer preferences.
Users can search in the way they find most convenient, leading to a smoother, frustration-free experience. It reduces the effort required to find products, enhancing overall user satisfaction.
Whether it’s typing, speaking, or uploading an image, customers can find and discover products throughout their search journey. This convenience fosters loyalty and repeat visits.
2. Enhanced Product Discovery
Customers can find products more easily with context-rich searches. For instance, pairing an uploaded image with more inputs narrows down the options significantly, making multimodal product discovery faster.
Building multimodal search and RAG (Retrieval-Augmented Generation) bridges the gap between customers' vague ideas and relevant products.
It allows retailers to surface hard-to-find or niche items, ensuring no product goes unnoticed.
3. Boosted Conversions
Customers are more likely to purchase when they find what they’re looking for quickly and accurately.
eCommerce multimodal search eliminates barriers to conversion by improving relevance and usability. By reducing the time to decision, it minimizes cart abandonment and boosts impulse purchases.
Additionally, personalized results encourage customers to explore complementary products, increasing average order value.
4. Inclusive Accessibility
This search technology supports diverse user needs. For instance, visually impaired users can rely on voice search, while others might use multimodal image search to bypass language barriers.
Mobile search with multimodal queries further supports users in multilingual or global markets, where language and mobile devices can otherwise be a barrier to entry.
By catering to everyone, businesses expand their customer base effortlessly.
5. Contextually Accurate Results
By analyzing multiple inputs and their relationships, multimodal RAG ensures results are tailored to the user’s specific intent, leading to higher satisfaction.
It reduces irrelevant results, saving customers time and effort. The system learns from user behavior to refine accuracy, providing ever-improving experiences.
This depth of understanding fosters their trust in the search process, as customers feel understood and valued.
What Are the Use Cases of Multimodal Search in eCommerce?
Here are some multimodal search use cases:
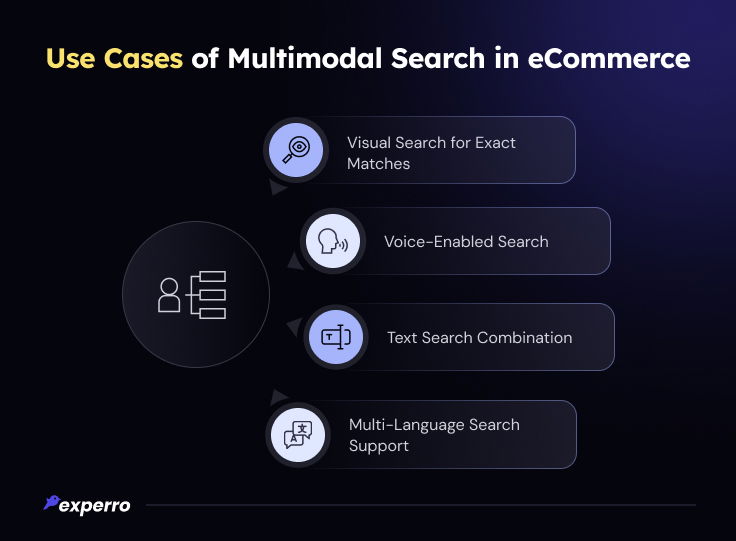
1. Visual Search for Exact Matches
Customers can upload images to find products with similar features. This is particularly useful for fashion and home decor, where visual details matter significantly.
For example, shoppers can upload photos of a dress they saw online or furniture that matches their home’s aesthetic.
Visual search via images reduces the need to describe products manually for the customer and accelerates the discovery process.
2. Voice-Enabled Search
Voice search allows users to describe what they’re looking for verbally. This is ideal for on-the-go shoppers or those who prefer hands-free interaction.
It is particularly helpful for complex queries, like “Find me a black jacket under $100 available in medium size”.
Voice-enabled searches also make eCommerce more inclusive for users with physical limitations.
3. Text Search Combination
Pairing text with image search or other inputs—like a voice command—provides more detailed and accurate results.
For example, searching for “red sneakers” while uploading a photo of a specific design. This combination creates a richer context, helping shoppers find exact matches faster.
It’s especially useful for niche items or when customers aren’t sure how to describe their desired product.
4. Multi-Language Search Support
By supporting searches in multiple languages, this technology ensures accessibility for global audiences, breaking down language barriers.
It allows retailers to cater to diverse markets, enhancing their international reach. Whether typing, speaking, or uploading images, users can search confidently in their preferred language, improving conversion rate optimization and inclusivity.
What Are the Key Challenges You Might Face in Adopting Multimodal Search?
Adopting multimodal eCommerce search comes with its own set of challenges.
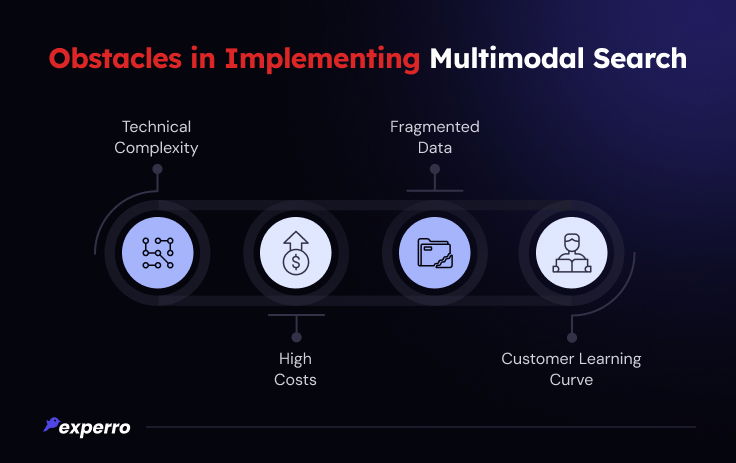
Let’s diagnose the challenges to perform multimodal search on your platform in the section below.
1. Technical Complexity
Integrating multiple technologies like NLP, image recognition, and voice processing requires significant technical expertise and resources. The complexity increases with the need to ensure smooth interoperability across these systems.
However, Experro’s platform seamlessly integrates AI-powered search and advanced technologies, simplifying the process and reducing technical overhead for businesses.
2. High Costs
The advanced infrastructure and development costs for implementing advanced search can be a barrier, especially for smaller businesses.
In this case, Experro offers a scalable solution that help businesses access multimodal AI search without the high upfront costs, making it affordable for both large and small businesses.
3. Fragmented Data
Ensuring data from various sources is unified and clean for processing can be difficult, particularly for businesses with legacy (old) systems. Poor data management can affect the accuracy of search results.
To address this, Experro’s AI-powered platform unifies fragmented data and ensures smooth integration, providing accurate, context-aware search results.
4. Customer Learning Curve
Introducing new search methods may require educating customers, as they might initially struggle to use the feature effectively. Customers may resist new multimodal search interfaces or technologies if they’re not familiar with them.
To help with this, Experro provides intuitive, user-friendly search interfaces that ensure smooth adoption, allowing customers to easily transition and engage with multimodal databases.
The global multimodal AI market is projected to reach $98.9 billion by 2037.
Transform Your eStore with Experro’s Next-Gen Multimodal Search Functionality!
Experro’s digital experience platform is built to streamline and elevate the multimodal search experience for your eCommerce business.
With AI-powered search at its core, the platform capabilities built-in advanced technologies like image recognition and real-time data processing to create a seamless and dynamic shopping journey.
The upcoming addition of voice command functionality will further enhance the customer experience.
By combining these capabilities, Experro ensures that your customers benefit from fast, accurate, and hyper-personalized search results, transforming your eStore into a more intuitive, customer-focused platform.
This ongoing innovation helps keep your business at the forefront of the eCommerce experience.
Conclusion
Multimodal search is reshaping how customers interact with eCommerce platforms, offering unmatched accuracy and ease.
By adopting it, businesses can stay ahead of the competition and deliver the seamless experiences today’s customers demand.
With Experro’s support, integrating eCommerce multimodal AI search is simple and effective. Contact us on a call to learn how we can help elevate your eCommerce platform with cutting-edge multimodal search capabilities.
FAQs
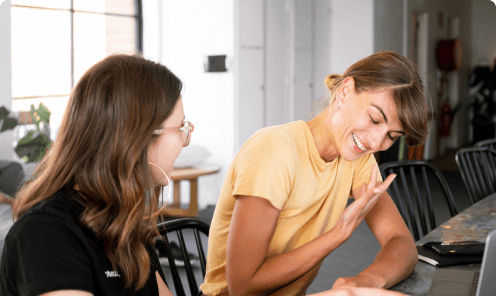
Pallavi Dadhich
31 January 2025Pallavi is an ambitious author known for her expertise in crafting compelling content across various domains. Beyond her professional pursuits, Pallavi is deeply passionate about continuous learning, often immersing herself in the latest industry trends. When not weaving words, she dedicates her time to mastering graphic design.