Role of eCommerce Search Algorithms: From Queries to Conversions!
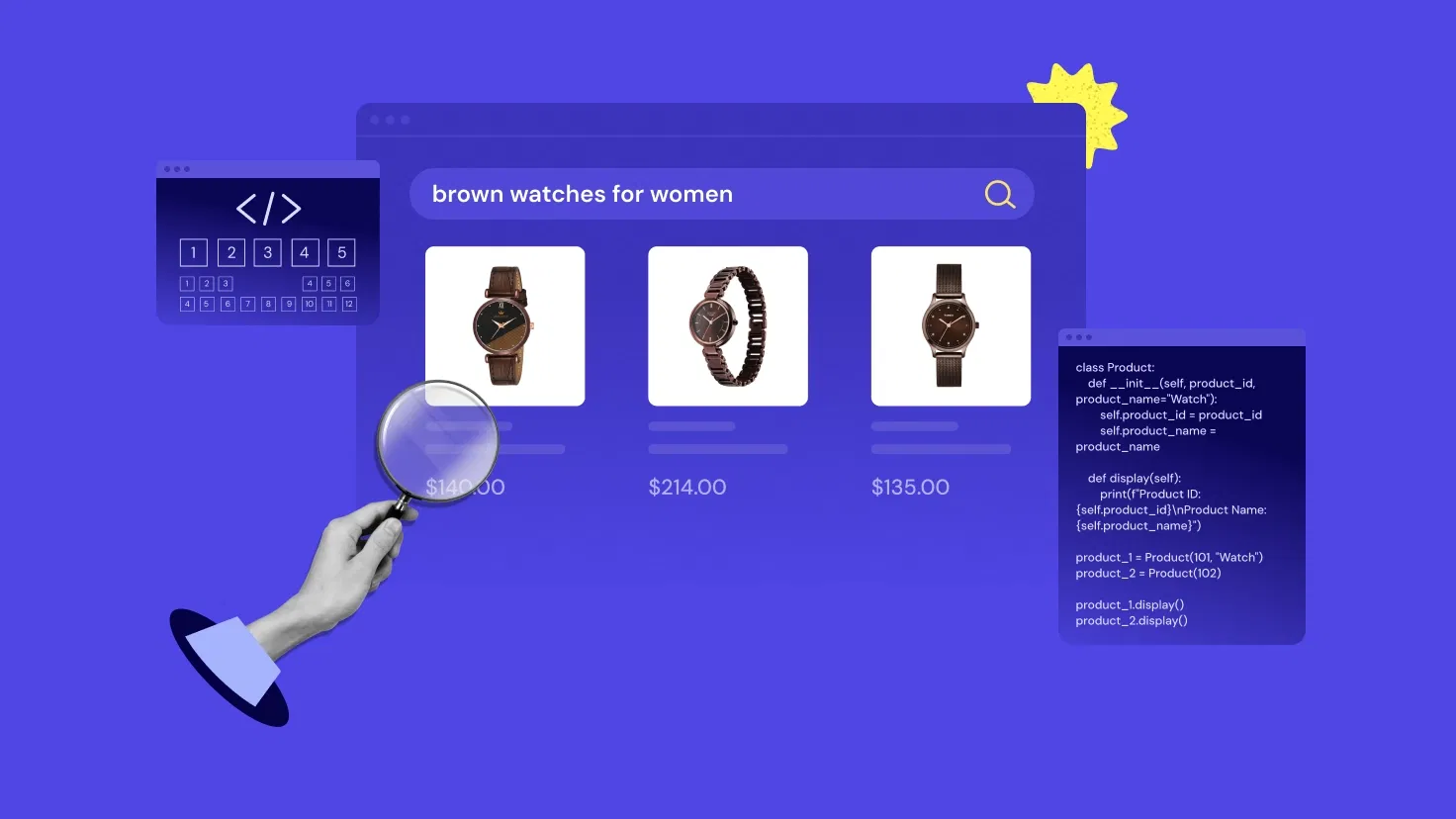
What’s Inside
- What Are eCommerce Search Algorithms?
- What is the Difference Between Search and Search Algorithm?
- How Do eCommerce Search Algorithms Work?
- Can Your eCommerce Search Engine Handles These Query Types?
- What Are the Core Components of Modern eCommerce Search Algorithms?
- Challenges in Implementing eCommerce Search Algorithms
- Optimize Your eCommerce Product Search Algorithm with Experro!
- Conclusion
Key Takeaways
- A well-optimized eCommerce search algorithm enhances product discovery, reduces zero-result searches, and improves conversions.
- AI-powered search adapts to user intent, corrects errors, and delivers personalized, context-aware results.
- Advanced eCommerce search algorithm ranking models, real-time indexing, and multimodal search improve relevance and shopping experience.
- Experro’s AI-powered eCommerce search algorithm can optimize relevance, speed, and personalization to drive higher conversions and engagement.
Nothing frustrates an online shopper more than searching for a product and getting irrelevant—or worse, zero results.
Every failed search is a lost sale.
But with the right eCommerce search algorithm, you can turn frustration into seamless discovery, driving more conversions and happier customers.
An intelligent search algorithm doesn’t just show results—it understands the intent behind queries, corrects mistakes, and personalizes experiences.
When done right, it becomes your biggest competitive advantage. In this blog, we’ll discuss how eCommerce search algorithms work and how you can leverage them for your business growth.
What Are eCommerce Search Algorithms?
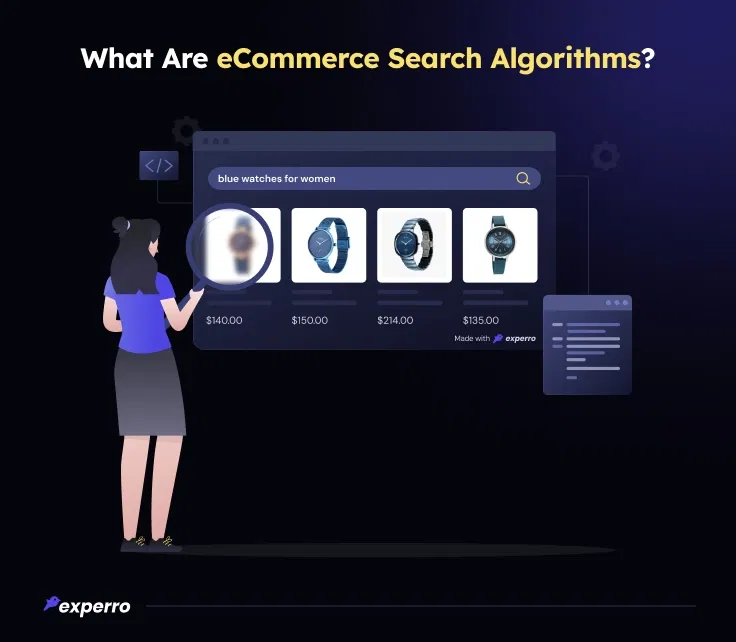
An eCommerce search algorithm is the engine behind your store’s search functionality. eCommerce search algorithm powers your store’s search functionality, processing user queries, matching them with product listings, and ranking results based on relevance.
A well-optimized content-based search algorithm ensures that customers find what they’re looking for—quickly and effortlessly.
Now that we understand how eCommerce search algorithm work, let’s break down the key differences between search and search algorithms.
What is the Difference Between Search and Search Algorithm?
A search is simply the act of finding information, like looking for a product in an online store.
On the other hand, a search algorithm is the intelligence behind it—it analyzes queries, matches keywords, and ranks results to deliver the most relevant answers efficiently.
Aspect | Search | Search Algorithm |
---|---|---|
Meaning | The act of finding specific data or information from a dataset. | A step-by-step method or logic used to retrieve information efficiently. |
Example | Searching for a contact in your phone by scrolling through the list, manually checking each name until you find the right one. | Using the search bar to type "Mir", which instantly shows results like "Mirror," by matching the typed letters, making the process faster. |
How Do eCommerce Search Algorithms Work?
A search engine on an eCommerce store follows a structured process (algorithm) to deliver accurate search results.
Here’s how the best eCommerce search algorithm work:
1. Query Processing
The algorithm analyzes what the customer is searching for - including keywords, intent, and context. It also detects spelling errors, synonyms, and alternative phrases to improve search relevance.
For example: Searching for ‘phone case’ also brings up ‘mobile cover’ by recognizing synonyms.
2. Indexing & Retrieval
It scans through product data to find the most relevant matches. The system creates an organized index that allows fast and efficient access to relevant product listings.
For Example: Just like a search bar in an online bookstore, it instantly finds all available editions of a novel.
3. Ranking & Sorting
The results are ranked based on various factors like relevance, popularity, and eCommerce personalization. Advanced ranking models also consider customer behavior, trends, and seasonal demands to improve sorting.
For example: A search for ‘winter jackets’ prioritizes those trending in colder regions.
4. Refinement & Learning
AI and ML continuously improve the site search experience based on user behavior. Over time, the system adapts to changing customer preferences and enhances result accuracy through automated learning.
For example: If most users searching ‘gaming laptop’ prefer high-refresh-rate screens, the system adapts and ranks those higher.
A powerful product engine algorithm does more than just return results; it helps customers discover products they didn’t even know they needed.
Can Your eCommerce Search Engine Handles These Query Types?
Customers search in different ways, and your platform’s search algorithm should be able to handle them all.
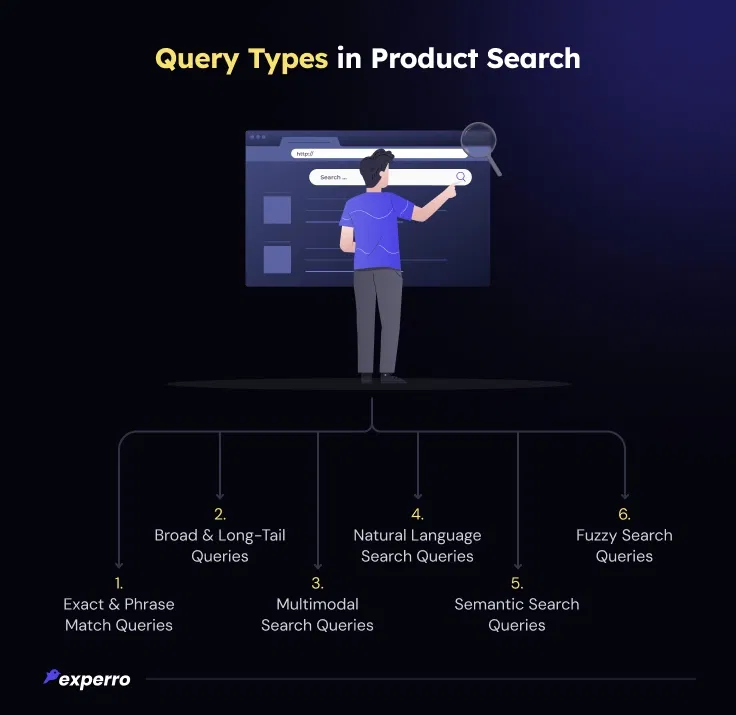
Here are the key query types to optimize for:
1. Exact & Phrase Match Queries
Customers expect to see results that match their search term exactly. Your search ranking algorithm should prioritize precision for these queries.
Example: Searching ‘gold ring’ should display all gold rings without irrelevant results such as ‘gold necklace’.
2. Broad & Long-Tail Queries
A customer may search for ‘women’s shoes’ (broad) or ‘black running shoes for women’ (long-tail). Your search intent algorithm should understand both and return the most relevant results.
3. Multimodal Search Queries
Shoppers increasingly use voice search and image-based searches. A visual search algorithm enables customers to upload photos, while voice search algorithm processes spoken queries.
4. Natural Language Search Queries
Shoppers tend to search in natural language like “What are the best noise-canceling headphones under $200?”). Advanced eCommerce algorithms should recognize intent and filter products accordingly.
5. Semantic Search Queries
A smart eCommerce algorithm should leverage semantic search to understand synonyms and related terms. A search for ‘running shoes’ should also return ‘sports sneakers' or ‘jogging shoes.’
6. Fuzzy Search Queries
Customers often make spelling mistakes. A real-time search algorithm uses fuzzy search to autocorrect and suggest the right products. If someone types ‘sneekers’, they should still see ‘sneakers’ in the search results.
Now that we’ve covered key query types, let’s dive into the essential components that power modern eCommerce search algorithm.
What Are the Core Components of Modern eCommerce Search Algorithms?
A well-optimized eCommerce web search algorithm consists of multiple layers working together to deliver accurate, relevant, and personalized results.
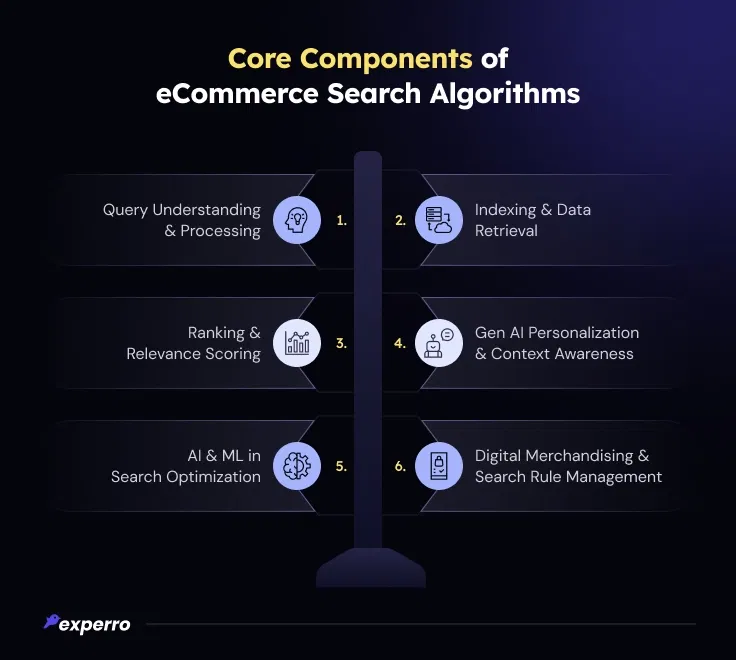
Let’s explore the core eCommerce search algorithm features that power a high-performing site search experience.
1. Query Understanding & Natural Language Processing
Search begins with understanding what the customer wants. Natural language processing (NLP) helps break down queries, identify intent-based search algorithm, and handle variations in phrasing.
Fuzzy search techniques correct spelling mistakes in real-time, ensuring users find relevant products even with typos.
Additionally, semantic search connects queries to related terms, improving result accuracy. By implementing auto-suggestions and predictive search, your business can enhance user experience and reduce search friction.
2. Indexing & Data Retrieval
A fast and efficient search process requires a well-structured indexing. Indexing organizes product data for quick retrieval, ensuring search results appear instantly. Inverted indexing techniques help locate relevant results efficiently.
Real-time indexing ensures that new products or price updates reflect instantly in gen AI-powered search results, preventing outdated information from affecting conversions.
Only AI-powered crawlers and data parsers can keep the index updated with the latest inventory changes.
3. Ranking & Relevance Scoring
Once results are retrieved, they must be ranked effectively. Relevance scoring algorithms prioritize products based on factors like keyword match, popularity, and user behavior and provide profile-based recommendations.
Weighted ranking models consider multiple data points, such as click-through rates, conversions, and stock availability, to display the best products first. Personalized ranking strategies use past interactions to tailor search results for individual shoppers.
Merchants can also apply business rules to influence ranking, ensuring high-margin or trending products get more visibility.
4. Gen AI Personalization & Context Awareness
AI-driven personalization adapts to each shopper’s unique preferences by analyzing browsing history, past purchases, and session behavior. This ensures more accurate and personalized search solutions.
On top of this, context-aware algorithms further enhance search relevance by considering real-time factors like location, device type, and seasonal trends. These dynamic adjustments help shoppers find what they need faster.
Additionally, conversational AI allows users to interact naturally with search, mimicking real dialogue. As AI continuously learns from these interactions, it refines personalization even further, delivering highly relevant recommendations tailored to each shopper.
5. Artificial Intelligence & Machine Learning in Search Optimization
Intent-based AI search understands user needs beyond exact keywords, delivering smarter results. Automated search tuning eliminates the need for manual adjustments, allowing algorithms to evolve based on real user behavior.
Machine learning plays a critical role in refining eCommerce product search algorithm accuracy over time. Self-learning algorithms analyze click-through rates, bounce rates, and purchase patterns to enhance ranking models.
90% of U.S. consumers consider a good eCommerce site search solution absolutely essential for their customer’s online shopping experience.
6. Digital Merchandising & Search Rule Management
Beyond accuracy, search should drive business goals. Personalized merchandising strategies allow your business to boost specific products based on promotions, profit margins, or inventory levels.
Search rule management enables merchants to fine-tune search behavior using business logic, ensuring priority products appear first. AI-powered dynamic ranking automatically adapts search functionality based on sales trends and engagement data.
Additionally, customized banner placements within search results can highlight featured collections, enhancing product visibility.
Challenges in Implementing eCommerce Search Algorithms
Building an effective eCommerce product search algorithm comes with its own set of challenges.
From handling complex queries to balancing personalization and performance, your eCommerce product search engine must navigate multiple obstacles to ensure a seamless search experience.
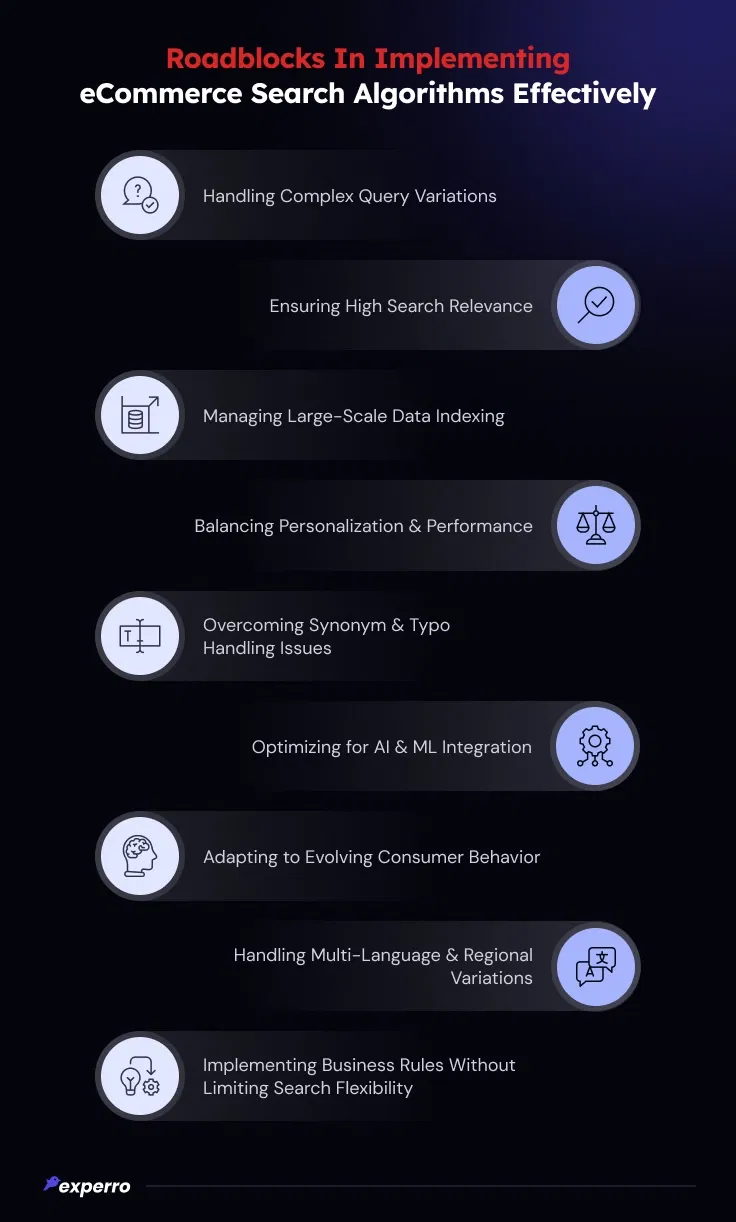
Here’s a breakdown of key challenges and how Experro helps solve them.
1. Handling Complex Query Variations
Shoppers use different phrasing, abbreviations, and even multi-word queries when searching. Traditional eCommerce search engines struggle with long-tail queries, complex terms, and conversational search patterns.
Without context-aware processing, users may receive irrelevant results, leading to frustration and drop-offs.
However, Experro’s AI-powered search understands natural language, allowing for more intuitive and accurate query matching.
2. Ensuring High Search Relevance
A search bar UI must display the most relevant products instantly. Poor ranking models lead to low engagement, high bounce rates, and lost sales.
Factors like user intent, seasonality, and trending products should be considered for better relevance.
To overcome this, Experro leverages AI-driven ranking and real-time behavioral data to refine search relevance, ensuring customers find what they need faster.
3. Managing Large-Scale Data Indexing
As product catalogs grow, maintaining fast and efficient indexing becomes critical.
Large datasets slow down retrieval times, leading to lagging search performance and outdated results. Keeping indexes updated across omnichannel eCommerce adds complexity.
In this case, Experro’s real-time indexing ensures products, prices, and stock updates reflect instantly in search results.
4. Balancing Personalization & Performance
A personalized search algorithm enhances user experience, but excessive customization can slow down search performance.
Processing large amounts of user data in real time requires robust AI models and efficient computing.
To tackle this, Experro provides personalization at a scale that balances traffic, speed and relevance, ensuring tailored search algorithm for product recommendations without performance lag.
5. Overcoming Synonym & Typo Handling Issues
Customers often misspell brand names or product terms, leading to zero results.
Without an intelligent synonym and typo-handling system, search engines fail to recognize variations of the same product, frustrating shoppers.
However, Experro’s fuzzy search and synonym recognition corrects typos and maps variations, ensuring better search accuracy.
6. Optimizing for AI & ML Integration
Gen AI-powered search requires constant learning and tuning to improve accuracy.
Many businesses lack the infrastructure to implement AI models effectively, resulting in outdated search results and rigid rule-based systems.
To address this, Experro’s built-in AI models auto-optimize search rankings, eliminating the need for manual fine-tuning.
7. Adapting to Evolving Consumer Behavior
User search behavior constantly changes with trends, seasons, and buying preferences.
Static search engines struggle to adapt to shifting demands, leading to outdated or irrelevant results.
In this case, Experro’s adaptive AI learns from user interactions, ensuring search results evolve with changing user preferences.
8. Handling Multi-Language & Regional Variations
Global eCommerce stores need multi-language support, but direct translations often fail to capture regional nuances.
Localized search requires cultural context, currency adaptations, and regional synonyms.
To address this, Experro provides multilingual search capabilities, ensuring accurate and culturally relevant results across different markets.
9. Implementing Business Rules Without Limiting Search Flexibility
Retailers want to boost specific products, but rigid business rules can disrupt natural search ranking.
Over-prioritizing promoted items may reduce search quality, affecting customer trust and engagement.
However, Experro allows digital merchandising rules, balancing business priorities while keeping search results relevant.
Now, let’s explore how Experro’s AI-powered algorithms can transform your eCommerce search experience.
Optimize Your eCommerce Product Search Algorithm with Experro!
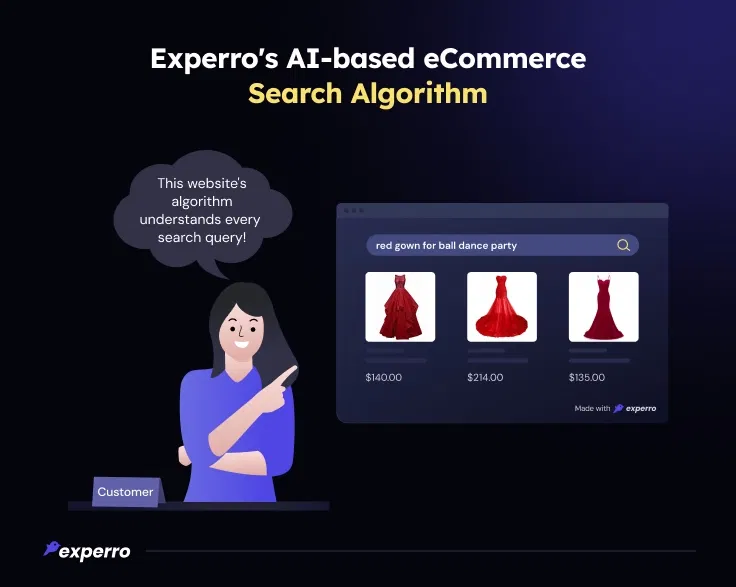
Experro’s AI-driven eCommerce product search algorithm ensure your customers find what they need fast.
Here’s what we offer:
✅ eCommerce Search – AI-powered search understands queries in real time for precise results.
✅ Personalized Search Results – Personalization tailors product suggestions based on browsing and purchase history.
✅ Voice & Visual Search Capabilities – Enables multimodal search experiences.
✅ Smart Merchandising – eCommerce merchandising lets you prioritize products strategically.
✅ Lightning-Fast Performance – AI-driven indexing ensures instant results.
Upgrade your search experience with AI-driven precision and speed. With Experro, every search leads to better engagement and higher conversions.
Conclusion
A well-optimized eCommerce search algorithm is a game-changer for online stores.
By leveraging AI, ML, and smart personalization, you can drive more conversions and keep customers engaged.
Investing in a powerful search experience ensures visitors turn into loyal buyers. Is your platform falling short of a few or all functionalities? Want to improve your ROI? Let’s discuss on a call!
FAQs
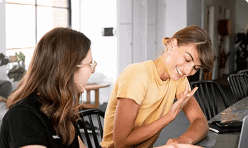
Pallavi Dadhich
13 March 2025Pallavi is an ambitious author known for her expertise in crafting compelling content across various domains. Beyond her professional pursuits, Pallavi is deeply passionate about continuous learning, often immersing herself in the latest industry trends. When not weaving words, she dedicates her time to mastering graphic design.